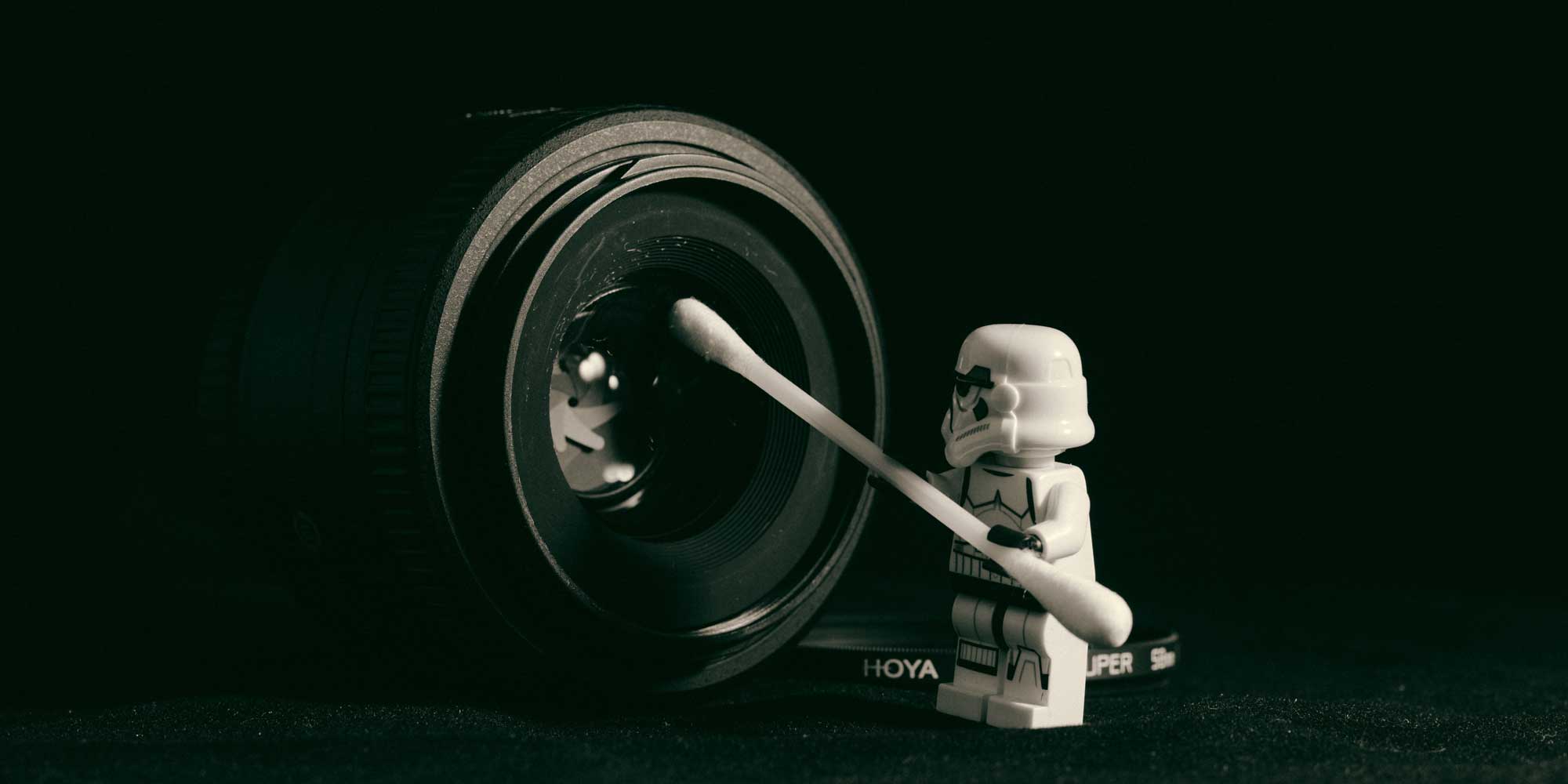
More and more tools available are to create models of IA, it has to make it easier for companies that power the machine for their applications Learning usable. What is the experience in the field if necessary for the implementation was facilitated by libraries tools and frameworks like Google tensorflow that arose.
To be clear, none of this is easy ', but it is quite possible that the most difficult part of the equation acquires AI and fight, perhaps most poignant, make the data cleaning required job.Engineers inexperienced AI and underestimated the necessary time and effort data to a to get the point where AI will achieve the greatest impact, where the model will be so strong and predictive as it can be.
We talked with many scientists and engineers dedicated to the feeling that in a particular project AI, parking, mobile (these records in size can be bulky), control and data organization includes frequency of 70% to 80% of the time to a project , Model implementation and design can often form the shortest backend employment.
In this sense, we have this idea AI move put experienced hands frmo together tips and tactics, cleaning and processing of data.
In short form (see below), our results:
- If the data is very large, sometimes it is the best way to move the algorithms, data not
- Even companies with massive databases owner time for AI massage
- Unstructured Data dark and can not be ignored
- It is better if you really look at it your data, the more the better
- Automating inspection - also includes AI in the process
- Embracing automation but do not automatically exclude Blanca
- Other models use artificial intelligence to analyze data coming
- Scroll through data, write and keep it out of the model
data movement
Large amounts of data can build transportation for complicated machine learning models, in some cases, may mean that a visit to the site is required. A terabyte or two slightly normal in all channels are moved, but things can be unpleasant if the data affect multiple petabytes or exabytes in question. At this point, the data must be physically transported.
Think about the best way to facilitate the movement of data while redundancy and the ability to ensure treat.
While intuition might suggest that more data is always better, not only difficult to do the treatment of large quantities because of the physical location of the data, but also requires time and processing power.
Using the Web on the computing power or transport can be expensive, such as cloud - computing - solutions can be prohibitively expensive to build models of IA, which is about wrote recently .
Sometimes it's better not to think about moving data, but to move the machine method, the units that analyze the data and to process the construction of the model.
"Advanced systems in general on the algorithms that move data rather than the data to move to the algorithms," said Siddhartha Agarwal, vice president of product management and strategy at Oracle.
Engineers should always try to use as much data as possible. AI algorithms are good at finding what is relevant and what is not, so it does not hurt err in providing more data for these algorithms. Incidental finding unknown correlations likely to occur if a model is set up with more information, not less, says Agarwal.
Even companies with massive databases owner time for AI - Massage
Most applications and databases in use today, especially those that are full of files are not threatened as it is today built with the specter of bird flu. It is therefore often applications allows non-standard data in their databases to write that in the expected plain text can be riddled with white, snacks, misspellings non-standard things like integers. This is not a big problem if the data should usually done in a relational database work, where he met in the rule in small groups or by themselves.
But when he shot and in a new place, be cast with new expectations about the engineers may find that their data assets, claiming to be so valuable, are not originally originally intended as loans IA shown.
A common problem for large companies with data cells that they have stored in silos, with little connective tissue fibers between the single database.
"Many companies use a seprate CRM platform, a platform for customer service and management platform e-mail campaign," said Chris Matty, CEO and co-founder of Versium that used for predictive analysis AI. "During all these platforms for the company's advantage, it's different systems that can cause several problems analytically."
Data silos can result in duplicate information, some of which match; some of which may contradict. Data silos can also limit a company's ability to make fast the lessons of their internal data.
This common circumstance companies and scientific data do more work data fusion to do and all the areas of agreement between them. This can be done with scripts to build large tables with assumptions, but builds these things take time and consideration those who know the best data.
Enter to implement the IA without the limits of the current practice of data collection and have so make sure its budget for these issues in a startup company or lead projects pass on the cost and time, especially with the existing records.
Most importantly, keep all these things to consider when building new applications and new data structures. Make sure that specific information now pave the way for an easier AI after analysis.
Dark unstructured data and should not be ignored
They are available in many shapes and forms of data. Gabriel Moreira, the lead scientific data CI & T, a digital agency, indicates that 80% of organizational data unstructured. These are such things as newspapers, documents, images and other media. This "dark" data is more difficult to analyze than structured data, they do not provide the necessary level of organization, and some of them can not in traditional databases selected data is stored.
"But just because it's harder not to analyze, not useless data mean," Moreira said. "In general, there are many hidden options in a haystack."
For example, Web server logs the routes can be used to understand user to a website to the model of the user settings and personalized recommendations can provide. The scanned documents can take pictures with OCR techniques are scanned and natural language processing can provide an overview of the processes available that have collected these documents. Call records are converted to text centers to analyze the main motivation of calls and conversations. Webcams in stores could be used to assess customer satisfaction, while the shelves and airports cameras surfing can be used to automatically detect suspicious behavior.
use this data requires special care and time and special processes that ensure data straight and clean. The process of translating analyze, and different data types organizing this for a task as it is likely to include much of the work of an experienced modelers AI, but the rewards are worth it.
The structured and unstructured data processing can lead to a higher degree of accuracy and usefulness to more powerful models. Building models in this way requires more care, more steps and more. But these are the types of buildings that can make a board AI entrepreneurs can be difficult and non-intuitive to other gaming companies.
View your data
This part of the mission of AI is not attractive. It is time, and often devours be spent on a particular project to 80% of the time.
A good starting point, recommends Amanda stent, the architect of the natural language processing Bloomberg, is currently looking for data, or at least a part of it.
Stent had a job at the beginning of his career was to identify the temporal order of events (whether an event occurred before or after or during the event B). a record exists, but the stent-team could not record any clear basis for this task using this data.
After a few weeks, the data is actually considered and found that the logical conclusion of the temporal relationship has not done in the data analysis or training - so that when an event took place in reality before the event B, data had not marked occurred as an event B by events on and so there is little chance that this relationship model was not found.
"Two weeks were entirely too long without having to go to the data to look for," said stent. "You crazy Make sure you look at your data at the beginning, before spending comes weeks with the style and function of the technical."
Data cleansing can also mean added. As mentioned project, all scheduling stent was that is required to clean the data, adding tags, if necessary. She worked with other data sets, however, that the patch was not so easy where the missing elements for some engineers to interpolate values needed and fill in appropriate fields. Some models can handle better than others do, but it is preferable to provide a range of integrity and quality.
"Garbage in, garbage out", wherein the stent.
Automating inspection - also includes AI in the process
Whenever possible, the engineers have to write scripts that can verify that the data correspond within the required specifications. The ripe fruit is easy to build here. This would ensure things like date, time and corresponding standard convetions postal codes. It is preferred that these scripting capabilities, so they can easily adapted some freedom to have them for the record. Its design allows engineers to reuse scripts and compile a library of methods that allow the processing of data more quickly and ultimately better models of AI were built with data that is cleaner and more relevant.
It can help build during this step in previous models AI. In fact, the engineers AI form to process the data and then returned AI. Construction of the first models and methods will be very difficult, but can be used over and over again, and strong future models are much more accurate help.
"The quality of the data on an excellent application for AI, and probably the only way ahead to move there, the bar," says Massimo Mascaro, director, data and data engineering at Intuit, maker QuickBooks and Turbo Tax.
Intuit uses AI for anomalies and outliers to search the data and identify elements for inspection. The next major step in this process is to solve problems automatically, which automatically repair the machine or decide to reject an abnormal data. Finally, the Inuit AI want to push in the user interface of its products, so that users can be invited immediately if you (usually taxes or income data, which can be painful to be wrong) seems wrong your input. AI gets inherently clean Intuit data to this position and keep the IRS breastfed.
In addition, automated methods in any toolbox Engineering AI, those who can create models of AI provided by the senior class of companies to which services should be used to offer TDaaS outsourced human eyeballs means to review the data and to help themselves, to create, at an affordable price. Find out what these companies are good and which are not good for the shading process that trial and error requires, however, those who do, and they maintain a process with cheaper tickets man, necessary reward when obtaining frequency data in the crease.
Embracing automation but do not automatically exclude Blanca
Sometimes a goal can mean neglect or error, but knows the given context, it may also mean that the user wants to say something else to leave an empty space. This is an important distinction, the whole series of data to be taken into account.
For dates of employment, mean an end date blank in the position of a person in a company often, they can still in this work, said Mark Goldin, Technical Director of Cornerstone OnDemand, a cloud platform for recruitment and personnel management.
However, a description blank for a training is simply lack the data. "Depending on the application, we can always use the data, this special value, throw lines with faulty data or a type of average of the data without taking on or place," says Goldin.
The company has built its own tool called Datascope Godlin inspection to determine the quality of a record. Produce an assessment of red, yellow or green in the ranking of the quality of the data and the amount each customer and each data source AI see it.
Christopher Steiner is a best - selling New York Times author of two books, the founder of ZRankings and co-founder of Aisle50 (YCS11) Groupon acquired in 2015.
Aucun commentaire:
Enregistrer un commentaire